Picture this: It’s 3 a.m., and while you’re catching a few extra winks, your company’s security system is hard at work—detecting, analyzing, and neutralizing threats before they even have a chance to knock on your digital door. Welcome to the era of AI-powered cybersecurity, where machine learning is turning the tables on cybercriminals by shifting security from reactive firefighting to proactive prevention.
In today’s volatile digital landscape, staying ahead of cyber threats is not just a luxury—it’s a necessity. For decades, security teams have been trapped in a reactive cycle, patching vulnerabilities after breaches occur. But with the advent of advanced machine learning algorithms, the game is changing. This post explores how AI is revolutionizing cybersecurity, transforming it from a reactive process into a proactive shield that anticipates and prevents attacks before they even materialize.
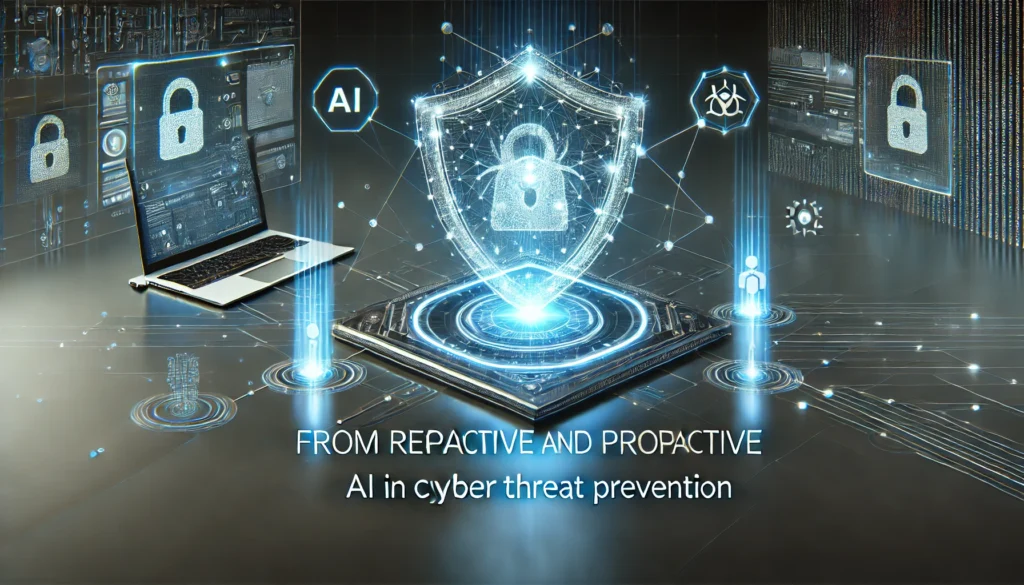
Introduction & Hook
Imagine the perfect security system: One that doesn’t wait for an intrusion to happen but instead forecasts potential threats with the precision of a weather forecaster predicting a storm. That’s the promise of AI in cyber threat prevention.
Why It Matters:
Traditional cybersecurity has always been about damage control—responding to incidents, containing breaches, and managing the fallout. But with cyberattacks growing more sophisticated and frequent, this reactive model is simply not enough. Machine learning is enabling a paradigm shift: instead of waiting for disaster to strike, organizations can now anticipate and prevent threats. This proactive approach not only minimizes risk but also saves millions in potential losses, making it a game-changer for senior leaders and IT decision-makers.
Background & Context
The Reactive Era of Cybersecurity
For decades, cybersecurity has operated in a reactive mode:
- Post-Breach Response:
Security teams are alerted after an attack has already penetrated defenses, forcing them to scramble to contain the damage. - Manual Intervention:
Analysts rely on logs and alerts, often drowning in a sea of data, to identify and mitigate threats after they occur. - Limited Predictive Capabilities:
Traditional systems are built on known threat signatures—making them vulnerable to new, emerging attack vectors.
Enter AI and Machine Learning
Artificial Intelligence (AI): At its core, AI is about enabling machines to learn, adapt, and make decisions. In cybersecurity, it means developing systems that can sift through massive amounts of data to detect anomalies, predict threats, and even neutralize them autonomously.
Machine Learning (ML): A subset of AI, ML involves algorithms that improve over time with exposure to new data. Rather than relying solely on pre-defined rules, ML models can identify patterns that signal potential threats—patterns that even the best human analysts might miss.
Why the Shift?
The exponential growth of data combined with increasingly sophisticated attack techniques has rendered traditional methods obsolete. Cybercriminals are evolving, and so must our defenses. AI-driven security transforms cybersecurity from a reactive “fix-it-after-the-fact” approach into a proactive system that stops threats in their tracks.
In-Depth Analysis: How Machine Learning Shifts Cybersecurity from Reaction to Prevention
Real-Time Threat Detection
Continuous Monitoring:
AI-powered systems operate around the clock, continuously analyzing network traffic, user behavior, and system logs. Instead of waiting for a breach alert, these systems detect subtle anomalies in real time.
Predictive Analytics:
By examining historical data and current trends, machine learning models can predict potential vulnerabilities. This foresight enables organizations to patch weaknesses before they’re exploited.
Example:
Consider a financial institution that uses AI to monitor its transaction data. Instead of detecting fraud only after suspicious activity occurs, the system identifies patterns that resemble known fraud behavior. It flags these transactions in real time, allowing the bank to intervene immediately—preventing losses and protecting customer trust.
Automated Incident Response
Faster Than Human Reaction:
When an anomaly is detected, AI can trigger automated responses in a fraction of a second—isolating affected systems, blocking suspicious IP addresses, or even rolling out temporary security protocols until human analysts can assess the situation.
Reduced Workload for Analysts:
By handling routine threat detection and response, AI frees up cybersecurity professionals to focus on complex investigations and strategic planning. This is crucial in an environment where the volume of alerts can easily overwhelm human teams.
Anecdote:
One global retailer integrated an AI-driven security platform to monitor its e-commerce operations. During a coordinated bot attack, the system automatically identified and isolated compromised nodes, preventing a full-scale breach. While the security team was alerted, the AI had already contained the threat, showcasing the power of automated incident response.
Enhanced Threat Intelligence
Learning from Every Attack:
Every security incident is a learning opportunity for AI systems. They continuously update their threat models based on new data, making them more effective over time. This iterative improvement is something traditional systems simply can’t match.
Global Collaboration:
Modern AI platforms often integrate threat intelligence feeds from multiple sources, including global cybersecurity networks. This means that when a new threat emerges in one part of the world, the AI can quickly adapt its defenses globally—an invaluable capability in our interconnected digital ecosystem.
Data-Driven Insights:
Organizations that deploy AI-powered security gain access to rich, actionable insights. Detailed analytics reveal not only what happened but why it happened, providing a roadmap for future prevention strategies.
The Paradigm Shift: From Fixing Breaches to Preventing Them
Traditional vs. AI-Driven Approaches:
Aspect | Traditional Cybersecurity | AI-Driven Cybersecurity |
---|---|---|
Response Mode | Reactive: Post-breach intervention | Proactive: Real-time threat detection & prevention |
Decision Making | Manual, slow, data-overloaded | Automated, rapid, data-driven |
Adaptability | Limited to known threats | Continually learns and adapts to new threats |
Analyst Burden | High—numerous alerts, manual triage | Reduced—automated, prioritized alerts |
This shift isn’t just about technology—it’s a fundamental change in how organizations view security. No longer are cybersecurity teams merely firefighters putting out blazes. They’re becoming strategists, using predictive insights to prevent those fires from starting in the first place.
Practical Takeaways & Actionable Tips
The transformation from reactive to proactive cybersecurity using AI isn’t just theoretical—it’s happening now. Here are some clear, actionable steps to help your organization make the leap:
Key Insights
- Invest in AI-Powered Security Solutions:
Look for platforms that offer real-time monitoring, predictive analytics, and automated response capabilities. - Prioritize Data Quality:
Effective machine learning depends on high-quality, comprehensive data. Ensure your data collection and management processes are robust. - Foster a Culture of Continuous Improvement:
Encourage your security team to adopt a learning mindset. Every incident should feed back into your AI models to enhance future performance.
Actionable Steps
- Conduct a Security Audit:
- Identify Gaps:
Review your current cybersecurity measures to identify areas where reactive approaches are failing. - Data Assessment:
Evaluate the quality and comprehensiveness of your data inputs—essential for effective AI performance.
- Identify Gaps:
- Implement Pilot Projects:
- Start Small:
Begin with AI solutions in specific areas, such as network monitoring or fraud detection. - Measure Impact:
Track the reduction in incident response times and the number of false positives to gauge success.
- Start Small:
- Train Your Team:
- Upskill Staff:
Invest in training for your cybersecurity professionals so they can effectively work alongside AI systems. - Cross-Functional Collaboration:
Encourage collaboration between IT, security, and data science teams to maximize the benefits of AI integration.
- Upskill Staff:
- Establish Continuous Feedback Loops:
- Iterate and Improve:
Use insights from every security incident to refine your AI models. - Regular Reviews:
Schedule periodic reviews of your cybersecurity strategy to adapt to emerging threats and technological advancements.
- Iterate and Improve:
- Engage with Industry Peers:
- Share Best Practices:
Participate in cybersecurity forums and conferences to stay updated on the latest trends and success stories. - Leverage Collective Intelligence:
Collaborate with other organizations to build a more resilient cybersecurity ecosystem.
- Share Best Practices:
Conclusion & Call-to-Action
The era of reactive cybersecurity is over. With AI and machine learning at the helm, businesses can shift from simply responding to threats to proactively preventing them. This is not a future scenario—it’s happening right now, reshaping the security landscape and redefining what it means to protect an organization in the digital age.
Recap of Key Points:
- Proactive Defense:
AI transforms security by predicting and neutralizing threats in real time. - Automated Response:
Machine learning enables rapid, automated actions that outpace human intervention. - Continuous Improvement:
Every incident is a learning opportunity, making AI-powered systems smarter and more effective over time.
Now, I want to hear from you. How is your organization leveraging AI to stay ahead of cyber threats? What challenges have you encountered, and what successes have you celebrated? Drop a comment below or join the conversation on social media. Your insights could be the spark that ignites further innovation in cyber threat prevention.
Embrace the shift from reactive to proactive—let AI be your first line of defense against cyber threats.